Cluster Analysis
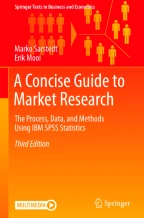
We provide comprehensive and advanced knowledge of cluster analysis knowledge. We first introduce the principles of cluster analysis and outline the steps and decisions involved. We discuss how to select appropriate clustering variables and subsequently introduce modern hierarchical and partitioning methods for cluster analysis, using simple examples to illustrate how they work. We also discuss the key measures of similarity and dissimilarity, and offer guidance on how to decide the number of clusters to extract from the data. Each step in a cluster analysis is subsequently linked to its execution in SPSS, thus enabling readers to analyze, chart, and validate the results. Interpretation of SPSS output can be difficult, but we make this easier by means of an annotated case study. We conclude with suggestions for further readings on the use, application, and interpretation of cluster analysis.
Electronic supplementary material
The online version of this chapter (https://doi.org/10.1007/978-3-662-56707-4_9) contains additional material that is available to authorized users. You can also download the “Springer Nature More Media App” from the iOS or Android App Store to stream the videos and scan the image containing the “Play button”.
This is a preview of subscription content, log in via an institution to check access.
Access this chapter
Subscribe and save
Springer+ Basic
€32.70 /Month
- Get 10 units per month
- Download Article/Chapter or eBook
- 1 Unit = 1 Article or 1 Chapter
- Cancel anytime
Buy Now
Price includes VAT (France)
eBook EUR 50.28 Price includes VAT (France)
Softcover Book EUR 63.29 Price includes VAT (France)
Hardcover Book EUR 89.66 Price includes VAT (France)
Tax calculation will be finalised at checkout
Purchases are for personal use only
Notes
Tonks (2009) provides a discussion of segment design and the choice of clustering variables in consumer markets.
See Arabie and Hubert (1994), Sheppard (1996), and Dolnicar and Grün (2009).
Whereas agglomerative methods have the large task of checking N·(N–1)/2 possible first combinations of observations (note that N represents the number of observations in the dataset), divisive methods have the almost impossible task of checking 2 ( N -1) –1 combinations.
There are many other matching coefficients, with exotic names such as Yule’s Q , Kulczynski , or Ochiai , which are also menu-accessible in SPSS. As most applications of cluster analysis rely on metric or ordinal data, we will not discuss these. See Wedel and Kamakura (2000) for more information on alternative matching coefficients.
See Punji and Stewart (1983) for additional information on this sequential approach.
The strong emphasis of gender in determining the solution supports prior research, which found that two-step clustering puts greater emphasis on categorical variables in the results computation (Bacher et al. 2004).
References
- Akaike, H. (1973). Information theory and an extension of the maximum likelihood principle. In B. N. Petrov & F. Csáki (Eds.), Selected papers of Hirotugu Akaike (pp. 199–213). New York: Springer. Google Scholar
- Arabie, P., & Hubert, L. (1994). Cluster analysis in marketing research. In R. P. Bagozzi (Ed.), Advanced methods in marketing research (pp. 160–189). Cambridge: Basil Blackwell & Mott, Ltd. Google Scholar
- Arthur, D., & Vassilvitskii, S. (2007). k-means++: The advantages of careful seeding. Proceedings of the 18th annual ACM-SIAM symposium on discrete algorithms. Society for Industrial and Applied Mathematics Philadelphia, PA, USA, pp. 1027–1035. Google Scholar
- Bacher, J., Wenzig, K., & Vogler, M. (2004). SPSS TwoStep Cluster – A first evaluation. Arbeits- und Diskussionspapiere/Universität Erlangen-Nürnberg, Sozialwissenschaftliches Institut, Lehrstuhl für Soziologie, 2004-2. http://www.ssoar.info/ssoar/handle/document/32715.
- Becker, J.-M., Ringle, C. M., Sarstedt, M., & Völckner, F. (2015). How collinearity affects mixture regression results. Marketing Letters, 26(4), 643–659. ArticleGoogle Scholar
- Caliński, T., & Harabasz, J. (1974). A dendrite method for cluster analysis. Communications in Statistics—Theory and Methods, 3(1), 1–27. ArticleGoogle Scholar
- Chiu, T., Fang, D., Chen, J., Wang, Y., & Jeris, C. (2001). A robust and scalable clustering algorithm for mixed type attributes in large database environment. Proceedings of the 7th ACM SIGKDD international conference in knowledge discovery and data mining. Association for Computing Machinery, San Francisco, CA, USA, pp. 263–268 Google Scholar
- Dolnicar, S. (2003). Using cluster analysis for market segmentation—typical misconceptions, established methodological weaknesses and some recommendations for improvement. Australasian Journal of Market Research, 11(2), 5–12. ArticleGoogle Scholar
- Dolnicar, S., & Grün, B. (2009). Challenging “factor-cluster segmentation”. Journal of Travel Research, 47(1), 63–71. ArticleGoogle Scholar
- Dolnicar, S., & Lazarevski, K. (2009). Methodological reasons for the theory/practice divide in market segmentation. Journal of Marketing Management, 25(3–4), 357–373. ArticleGoogle Scholar
- Dolnicar, S., Grün, B., Leisch, F., & Schmidt, F. (2014). Required sample sizes for data-driven market segmentation analyses in tourism. Journal of Travel Research, 53(3), 296–306. ArticleGoogle Scholar
- Dolnicar, S., Grün, B., & Leisch, F. (2016). Increasing sample size compensates for data problems in segmentation studies. Journal of Business Research, 69(2), 992–999. ArticleGoogle Scholar
- Kaufman, L., & Rousseeuw, P. J. (2005). Finding groups in data. An introduction to cluster analysis. Hoboken, NY: Wiley. Google Scholar
- Kotler, P., & Keller, K. L. (2015). Marketing management (15th ed.). Upper Saddle River, NJ: Prentice Hall. Google Scholar
- Lilien, G. L., & Rangaswamy, A. (2004). Marketing engineering. Computer-assisted marketing analysis and planning (2nd ed.). Bloomington: Trafford Publishing. Google Scholar
- Milligan, G. W., & Cooper, M. (1988). A study of variable standardization. Journal of Classification, 5(2), 181–204. ArticleGoogle Scholar
- Park, H.-S., & Jun, C.-H. (2009). A simple and fast algorithm for K-medoids clustering. Expert Systems with Applications, 36(2), 3336–3341. ArticleGoogle Scholar
- Punj, G., & Stewart, D. W. (1983). Cluster analysis in marketing research: Review and suggestions for application. Journal of Marketing Research, 20(2), 134–148. ArticleGoogle Scholar
- Qiu, W., & Joe, H. (2009). clusterGeneration: Random cluster generation (with specified degree of separation). R package version 1.2.7. https://cran.r-project.org/web/packages/clusterGeneration/clusterGeneration.pdf. Accessed 04 May 2018.
- Roberts, J. H., Kayande, U. K., & Stemersch, S. (2014). From academic research to marketing practice: Exploring the marketing science value chain. International Journal of Research in Marketing, 31(2), 127–140. ArticleGoogle Scholar
- Schwarz, G. (1978). Estimating the dimension of a model. The Annals of Statistics, 6(2), 461–464. ArticleGoogle Scholar
- Sheppard, A. (1996). The sequence of factor analysis and cluster analysis: Differences in segmentation and dimensionality through the use of raw and factor scores. Tourism Analysis, 1, 49–57. Google Scholar
- Tonks, D. G. (2009). Validity and the design of market segments. Journal of Marketing Management, 25(3/4), 341–356. ArticleGoogle Scholar
- Wedel, M., & Kamakura, W. A. (2000). Market segmentation: Conceptual and methodological foundations (2nd ed.). Boston, NJ: Kluwer Academic. BookGoogle Scholar
- Van Der Kloot, W. A., Spaans, A. M. J., & Heinser, W. J. (2005). Instability of hierarchical cluster analysis due to input order of the data: The PermuCLUSTER solution. Psychological Methods, 10(4), 468–476. ArticleGoogle Scholar
Further Reading
- Bottomley, P., & Nairn, A. (2004). Blinded by science: The managerial consequences of inadequately validated cluster analysis solutions. International Journal of Market Research, 46(2), 171–187. ArticleGoogle Scholar
- Dolnicar, S., Grün, B., & Leisch, F. (2016). Increasing sample size compensates for data problems in segmentation studies. Journal of Business Research, 69(2), 992–999. ArticleGoogle Scholar
- Dolnicar, S., & Leisch, F. (2017). Using segment level stability to select target segments in data-driven market segmentation studies. Marketing Letters, 28(3), 423–436. ArticleGoogle Scholar
- Ernst, D., & Dolnicar, S. (2017). How to avoid random market segmentation solutions. Journal of Travel Research, 57(1), 69–82. ArticleGoogle Scholar
- Punj, G., & Stewart, D. W. (1983). Cluster analysis in marketing research: Review and suggestions for application. Journal of Marketing Research, 20(2), 134–148. ArticleGoogle Scholar
- Romesburg, C. (2004). Cluster analysis for researchers. Morrisville: Lulu Press. Google Scholar
- Wedel, M., & Kamakura, W. A. (2000). Market segmentation: Conceptual and methodological foundations (2nd ed.). Boston: Kluwer Academic. BookGoogle Scholar
Author information
Authors and Affiliations
- Faculty of Economics and Management, Otto-von-Guericke- University Magdeburg, Magdeburg, Germany Marko Sarstedt
- Department of Management and Marketing, The University of Melbourne, Parkville, VIC, Australia Erik Mooi